In Dr. Strangelove, Kubrick plays with the absurdity of hyper sophisticated, immensely destructive deterrents of war strategy. The lunacy of commitment escalation gives birth to a machine able to blindly blow up the world following an even irrelevant misstep. Kubrick exposes the irrationality of such incentives, the convoluted nature of over-engineering, the tunnel-vision of mechanism design, and the dominant impact of widespread ignorance. You can’t fight in a war room, says someone in the movie, in an absurd attempt at preserving protocol in the shadow of mass extinction. This is not entirely fiction; the Doomsday Machine, the credible threat that could halt all conflicts, has been a useful absurdity for nuclear decades. It has worked, but at what cost. Apocalyptic tail risk should always be unacceptable, even on a probability-adjusted basis. But so we beat on, nukes against the current, borne back ceaselessly into the fear of annihilation.
Threatening to obliterate everything against a dishonest challenge might make you feel safe, but it works only for two kinds of worlds: one populated by the reckless or a desolate wasteland. Designers of the abstract should remind themselves of that.
Wisdom of the Wallet
We want to believe in magic. Even when we deny it, unexplained phenomena stimulate the brain. It doesn’t matter that we’ll soon adapt to a familiar reality, the suspense of the unknown remains exhilarating. The urge might well be rooted in our drive to expand our genetic pool through mating: seeking the new, the unfamiliar, increases the chances of survival for our lineage. Not even academics are immune.
One of their favourite seemingly magical phenomena is the so-called wisdom of the crowd, the almost magical ability to reach surprisingly accurate conclusions through the aggregation of unconnected and uninformed individual guesses. Galton’s 1907 ox-weight experiment is one of the most notable examples. At a country fair, 800 people were asked to estimate the weight of an ox after slaughter; the average of their guesses came to 1,197 pounds, remarkably close to the actual weight of 1,198. The dream of turning crap into gold has been with us for a long time, under many names. Still, it’s true that educated judgment aggregation works well in many contexts, with or without a financial angle.
Wisdom of the crowd, however, seems to work especially well when a financial overlay gets added to it. The debate between psychologists and economists on the usefulness of self-declared beliefs has been ongoing for years, with psychologists tending to take beliefs at face value, while economists focus more on the actual behaviours and actions of those same individuals.
Prediction markets → Prediction markets have been instrumental for crossing the chasm between economists and psychologists. In prediction markets, a contract is created that pays a fixed amount if the event occurs, allowing people to trade the contract by submitting buy and sell prices, similar to stock markets. In theory, the price at which the contract trades at any given time should reflect the market’s collective probability estimate of the event happening. Assuming a contract that pays $0 if the event does not occur and $100 if it does, and assuming risk neutrality, a spot price of $60 suggests the crowd estimates a 60% probability of the event occurring.
Prediction markets have consistently outperformed simple polling over the years, suggesting a win for the economists over the psychologists. The wisdom of the wallet appears to surpass that of the crowd. Psychologists, however, have argued that the superior performance of prediction markets may be due to the process of information aggregation. A price, rather than being a simple average of prior opinions, reflects the precise probability a marginal trader is willing to support at a given moment. Prediction prices evolve differently from trailing averages, being less influenced by the inertia and more responsive to external events. Research in the field supports this view—Dana, Atanasov, Tetlock, Mellers, 2019.
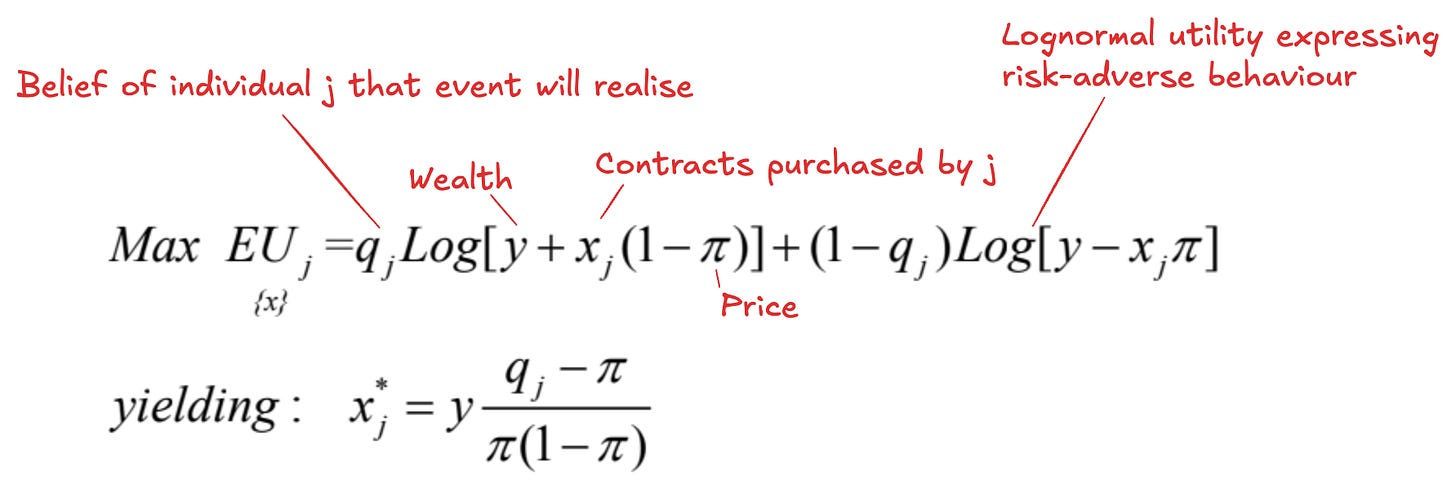
Prediction markets, indeed, seem to work. But we should resist again the urge to see them as magic and instead focus on understanding the market structure conditions that allow equilibrium prices to serve as unbiased estimates of event probabilities.
Market Equilibrium vs. Individual Preferences
Prices in prediction (and any) markets reflect the equilibrium between supply and demand, which itself is the aggregation of individual supply and demand preferences. In other words, individual asset demands directly influence market equilibrium. This relationship is particularly odd if we assume that, at least under risk neutrality, individual asset demand is a step function: i.e. if a contract’s market price is lower than the believed probability of an event occurring, a rational individual agent would invest 100% of her wealth in the contract. Significant research has been dedicated to understanding the conditions under which prediction market pricing can fairly and efficiently aggregate individual beliefs—see Risk Aversion, Beliefs, and Prediction Market Equilibrium and Interpreting Prediction Market Prices as Probabilities.
In modelling, individual asset demands are impacted by a few factors, including:
Individual coefficients of relative risk aversion—which may vary for participants
Joint distribution of beliefs—easily modelled through density functions
Initial wealth levels for each individual—initial i.e. ahead of the prediction game
Skipping the math, market structure modelling offers some key insights that are valuable for market designers and observers:
Market effectiveness. Prediction markets seem to be indeed highly effective at aggregating information. With normal risk aversion, in consistency with empirical estimates, and in cases where traders have logarithmic utility functions, equilibrium prices well reflect the mean of the belief distribution, regardless of how beliefs are spread out among participants
Positive impact of normal risk aversion. Under more restrictive conditions on the distribution of beliefs, the bias in the equilibrium price relative to the mean belief diminishes as the coefficient of relative risk aversion approaches one. Prediction markets targeted to extremely risk-seeking, or somehow oddly constructed and small communities, might not be well-functioning predictors
Long-shot bias. In a somewhat puzzling way, even with risk aversion present, prediction prices tend to overestimate the probability of events at the fringe of the probability distribution. This phenomenon aligns with modelling, as long as risk aversion is moderate and the belief distribution is symmetric. More extreme levels of risk seeking or aversion leads to even more polarised predictions
Wealth dependency. Relaxing the assumption that wealth levels are independent of beliefs leads to market prices becoming a wealth-weighted average of traders’ beliefs. If we assume that over the long run those with a history of accurate evaluations become wealthier, then those wealth-weighted averages are actually better predictors than unweighted averages
Why Do We Care?
But why should we ultimately care whether prediction market prices offer a fair representation of events probabilities? The implications go far beyond merely designing a balanced gambling game—they touch on sustainable market structure as well as deeper, even philosophical, matters.
Systematic decision-making. First, if prediction markets accurately reflect probabilities, they become invaluable sources of information for decision-makers across various fields, transforming these prices into powerful tools for informed (or algorithmic) decision-making.
Market expansion. Second, and perhaps more intriguingly, they introduce a novel avenue for creating financial instruments based on event-driven risks that diverge from traditional equity-like exposures, thereby enriching the landscape of financial markets with new forms of volatility.
Money can be viewed as a measure of entropy. The greater the (fairly measurable) entropy within a system, the more money can be created. To illustrate, imagine a financial system consisting of a single person and a single product—mortgages. The longer the person’s life expectancy, the larger the potential mortgage and, consequently, the greater the monetary supply in the system. In other words, the money available today reflects expectations that a range of future possibilities could generate sufficient value to repay the sum, adjusted for risk and present value. The larger the expectation funnel, the higher the current monetary base. Time is just one dimension along which entropy increases; information theorists define entropy as a measure of random loss of information: the further an observer is removed from a specific point in space-time, the higher the entropy levels.
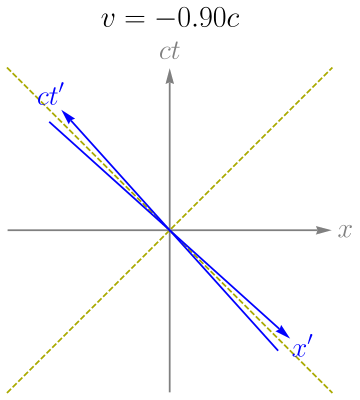
Financial markets, and more specifically crypto markets, have long been plagued by a high degree of autocorrelation, which has constrained their sustainable growth beyond a certain size. Markets have long sought uncorrelated sources of uncertainty, offering significant compensation to fund managers who can consistently deliver (read absolute) returns that are not significantly tied to the dominant risk drivers shaping financial markets. Events that are uncorrelated, or at least loosely correlated, with these major drivers (such as weather patterns, election outcomes, sports results, or life expectancies) could dramatically expand the collateral pool and the overall size of financial markets, much like the transformative impact of derivatives.
Systematising and financialising uncorrelated event probabilities is the shit.
Ethereum’s Love Story With Prediction Markets
Prediction markets have long been touted as one of Ethereum’s most promising use cases, owing to their dual utility: first, as a decentralised tool for aggregating information and forecasting future events, and second, for their capacity to seamlessly integrate and automate this data within the Ethereum ecosystem. Vitalik Buterin has been examining their role in the decentralised economy since Ethereum’s early days, highlighting their transformative potential— see 2014 and 2021. In 2018, it was the Augur project initiating the race to build a decentralised oracle and prediction market platform on Ethereum—whitepaper.
In Augur’s initial design, anyone could create a market around any (exogenous) event, with traders able to participate immediately after its creation. At some point in the future, Augur’s oracle would help verify the event’s outcome, enabling the smart contract to settle and distribute payouts to the winning traders. Beyond the aesthetics of blockchain-based market automation and the ability to apply itself to event-driven rather than perpetual asset-driven markets, Augur’s innovation centred on the decentralised oracle mechanism to solve disputes in a distributed manner and based on financial incentives.
Augur’s architecture is inherently complex, and as with all incentive design, the challenge lies in the intricacies of design and parameterisation. The core of Augur’s effort is to implement a robust mechanism design that incentivises crowdsourced oracle reporting for specific events. The (simplified) chart below outlines the key milestones of this process, which can be described as follows at a high level.
(1) Market creation. A market is created, with the creator defining the event and its window, and setting parameters to incentivise a timely reporting of the outcome. These parameters include the Designated Reporter, a Resolution Source, and various bonds such as Validity and No-Show Bonds. For as much as the elegance is the game-theoretical incentive design, it is ultimately in the interest of Augur and its users that correct outcomes are promptly reported and markets settle smoothly.
(2) Trading. Once created, the market enters its trading phase—the core stage. During this phase traders can buy and sell a complete set of shares using (interestingly) an order book mechanism. This is the most useful phase for Augur, allowing event-related price discovery.
(3) Reporting. When the event date has passed, the Designated Reporter is expected to report the outcome. Regardless of whether the Designated Reporter reports, the market eventually enters a dispute phase where others can challenge the reported outcome by staking an economic interest.
(4) Disputing. During a dispute, $REP (more on this to follow) holders can stake their $REP in support of an outcome different from the initially reported one. A challenge is successful if the staked $REP exceeds a specific Dispute Bond Size, i.e. a threshold which depends on the total $REP staked in that specific market and the distribution of stakes between the originally reported and the challenging outcomes. In essence, the more capital staked in the market, the harder it is to overturn the reported outcome—unless substantial stakes back disputing outcomes. Without getting into details, the core point is for financial actors to be incentivised to invest financial resources in $REP and to put $REP at risk for specific digital arbitration procedures.
(5a) Finalising. A market enters its final stage if it passes through a 7-day dispute round without having its tentative outcome successfully disputed, or after completion of a fork—below.
(5b) Forking. If, instead, a dispute succeeds, the market may either enter another dispute round or trigger a Fork state, depending on the total staked $REP. A Fork creates two parallel universes, splitting Augur’s genesis universe into separate sub-verses. $REP holders in the original universe must migrate to one of these new universes if they wish to continue participating and being financially exposed to new activity. Migration, bear in mind, is irreversible.
Prediction Markets’ Original Sins
In its first month, Augur attracted c. $1.5m of capital staked across over 800 outcome bets, indicating strong initial traction, but also early signs of harmful liquidity fragmentation. Over time, however, adoption remained niche, with activity levels struggling to match those seen in more traditional markets like CFDs and sports betting.
The performance of Augur offers a broader insight into the design and limitations of decentralised prediction markets—aka decentralised oracles.
Liquidity fragmentation. In proof-of-stake models, the integrity of the output is tightly linked to the capital at stake. When liquidity is dispersed across numerous, often redundant markets, it weakens the overall robustness of the oracle, making it more susceptible to manipulation and reducing the reliability of its outcomes.
Complexity and user engagement. When combined with intricate designs spanning market creators, outcome traders, reporters, and stakers, liquidity fragmentation introduces friction that significantly harm engagement from the outset. This isn’t specific to Augur and reflects a general crypto issue. The elegance and sophistication of some mechanism designs have often relegated them to the status of intellectual experiments rather than scalable solutions.
Fragility towards fraud and manipulation. The structural openness of decentralised oracles, designed to handle a broad and variable set of measurable arguments underpinned by financial incentives, creates a vast attack surface for fraud and manipulation. Proof-of-stake systems, though not unique to crypto, generally function only when the community is sufficiently large and cohesive, focused on binary decisions with limited paths for divergence—“I agree with this state of the world” or “I don’t”. While continuous forking has been explored by mechanism designers—including myself at least here and here, its practical application remains elusive when deployed in uncontrolled environments.
Capital inefficiency. When thinking in dollar terms, obtaining directional exposure through those prediction markets could be significant capital inefficient, reducing the effective risk-adjusted returns that take into account directional but also technological risk. This was particularly true during Augur’s early days, when tokenised deposits and other forms of dollar-backed stablecoins were not so common.
Things changed, at least temporarily, in the middle of 2020. There are only a few things as polarising as the (binary and global) US presidential elections, and the 2020 elections marked a turning point in liquidity aggregation for crypto prediction markets. Catnip, a simple frontend integrating Augur and Balancer, managed to drive daily open interest new inflow on Augur for $1m. Vitalik, monitoring the situation closely, took positions by executing a sophisticated arbitrage strategy to source the necessary $DAI liquidity and exploit the spread differences between Augur and Balancer. The trade structure, clearly outlined by Vitalik, became a wonderful essay on the problems we have outlined above.
Beyond Augur, another protocol started benefiting from the 2020 US election liquidity aggregation phenomenon, a less known newcomer in the space: Polymarket.
The Rise of Polymarket
Polymarket emerged leveraging lessons from Augur but trying to address its limitations. The project prioritised UX, opting for Polygon to cut transaction costs, and a more accessible, simpler interface with faster settlement times. Instead of Augur’s complex REP-based dispute mechanism, Polymarket employed a pragmatic, straightforward oracle solution using UMA’s Optimistic Oracle, sacrificing some elegance for efficiency.
Polymarket’s team built on Augur’s framework, prioritising a more pragmatic solution to the main pain points faced by its predecessor. UMA’s Optimistic Oracle plays now a central role, offering more rapid dispute resolution that prevents the protocol-disruptive forking risks Augur struggled with. Originally designed for synthetic assets and derivatives, UMA’s oracle has since expanded into a versatile dispute mechanism, allowing Polymarket to bypass the need for complex market creation and outcome verification processes. This partnership with UMA has freed Polymarket to focus on refining the UX and streamlining the user journey, making it difficult to consider Polymarket a direct successor of Augur.
UMA Optimistic Oracles are a more versatile oracle solution designed to bring arbitrary data on-chain in a decentralised manner. By operating based on the principle of optimistic resolution, most data requests are expected to be settled without dispute given that participants are economically incentivised to act honestly.
The decision tree below describes at a very high level the settlement mechanism. Compared to Augur’s oracle, UMA’s design is significantly simpler—and faster:
Proposers submit initial value responses, as well as a value bond as collateral to guarantee their honesty
Challengers can dispute the proposed value during a specific period, by bonding another value as collateral
Voters can vote their $UMA tokens on disputed value for a final resolution, with the protocol providing an incentive to $UMA stakers to vote with the majority
In a one-shot game, a Nash Equilibrium is achieved when:
Proposers submit a truthful value because deviating would lead to a high probability of being successfully challenged and losing the bonds
Challengers will only challenge if they have high confidence that the submitted value is false, and that the majority of the $UMA holders is honest
Voters are expected to vote truthfully as a Schelling point strategy, where the optimal strategy is to align with what other honest voters are expected to vote
From a (simplistic) mathematical point of view, the possibility to achieve a NE for the UMA game depends strongly on probability of a successful challenge. Skipping the intermediate steps for the busy ones, assuming a simultaneous game with perfect information:
Assuming that a rational challenger would challenge only if challenging is a dominant strategy—leading to a successful outcome, we can assume that looking at the probability of a challenge and the probability of a successful challenge is pretty much the same. Based on the formula above, the probability of a challenge depends basically on the combinatory effect of the proportional honesty of actors, and the challenger reward vs. the cost of challenging. The value of $UMA should not affect the dominant behaviour of the game.
Ultimately, the robustness of the oracle is proportional to the cost it would take any actor to amass a dishonest position vs. the potential benefit. Basically the importance of the bets settled through UMA should be proportional to the market value of the token. As a reference, currently $UMA has a market cap of c. $215m—vs. open interest for c. $165m on Polymarket globally. Not a great view.
Despite Polymarket’s upgrades, it struggled to maintain momentum—this until the 2024 US elections. Prediction markets, it turns out, tend to wake up every four years. 2024 saw volumes exploding ((Dune) from $6 million to $25 million, then $100 million, and now surpassing $650 million monthly. Over 80% of this activity remains tied to election-related markets, turning Polymarket into a temporary darling for political analysts and even a data reference for Elon Musk.
But the question looms: can it endure the post-election hangover? Polymarket’s competitive edge lies in its (kind of) decentralised nature, skirting around traditional regulatory barriers that shackle mainstream betting platforms, especially for political events. The CFTC’s 2022 action (a $1.4m fine) was a reminder that regulatory arbitrage has limits. And despite geofencing and formal restrictions, a substantial portion of the site’s traffic likely still comes from the US.
The US Elections Hangover: Life After Trump vs. Biden-Kamala-?
What’s the real deal behind prediction markets → If not managed appropriately, Polymarket’s pragmatism could easily become its main weakness. Betting, even online betting, is old news. The true innovation in decentralised markets isn’t the (temporary) ability to dodge the need for a state-mandated license. It’s in delivering censorship-resistant price discovery for binary events, unlocking composability across financial instruments, and expanding the financial universe to encompass previously inaccessible sources of volatility.
Think about it: entire insurance markets still depend on a centuries-old institution like Lloyd’s of London instead of relying on the seamless, automated settlements smart contracts could provide. Sure, algorithmic execution might carry some technological risks, but it’s hard to argue that these outweigh the risks posed by a room full of hungover underwriters every Friday morning.
The future of prediction markets hinges on addressing a few core challenges, some spanning beyond Polymarket and touching the broader crypto ecosystem:
Innovate oracle incentives: strengthen participation at the oracle level to enhance staking reliability through financial innovation
Promote oracle centralisation: minimising oracle fragmentation would benefit reliability—value fragmentation weakens integrity in proof-of-stake systems
Improve user engagement: create tailored/ gamified experiences that abstract the complexity of consensus mechanism, maybe focusing next on sports betting and insurance
Embrace Composability: leverage prediction market insights for downstream applications, maybe hoping for a revamp of inflows into DeFi
Curiously enough, it might be the election outcome itself that determines the post-election trajectory for prediction markets and crypto. After all, betting on your own demise can sometimes be a perfectly rational hedging strategy.